Propensity to Default Model for Online Credit Marketplace
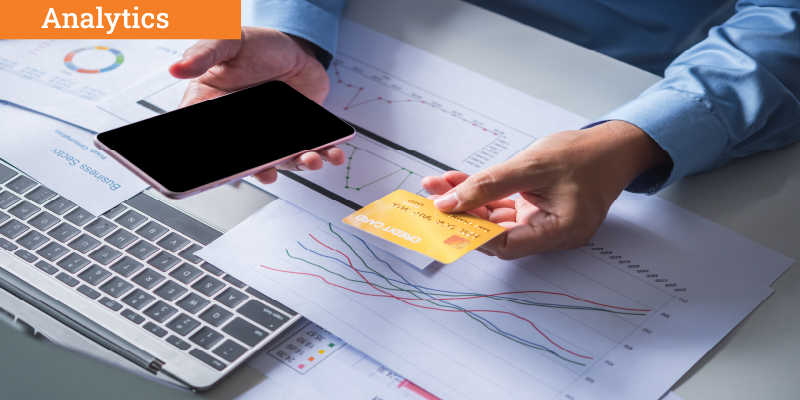
Abstract
Default risk is the chance that companies or individuals will be unable to make the required payments on their debt obligations. Lenders and investors are exposed to default risk in virtually all forms of credit extensions. In the event of a default, lenders may lose out on periodic interest payments and in many cases, the entire principal amount. Our objective of this study is to build a ‘Propensity to Default’ model which can predict the probability of default. We have also tried to understand the important variables that explain the default behaviour. For the purpose of our study, we have used the loan approval data of Lending Club, an online credit marketplace. Lending Club is the world’s largest online credit marketplace, facilitating personal loans, business loans, and financing for elective medical procedures. The company operates fully online with no branch infrastructure, and use technology to lower the cost. The predictive models are built on the actual data collected on the customers of this lending platform over a period of 4 years (2007 – 2011). We have used various Python libraries to perform detailed Exploratory Data Analysis (EDA) to display the vital statistics of different features. We have also built multiple models (Logistic Regression, Random Forest, Deep Learning etc.) to compare and select the most appropriate and accurate model. These predictive models can be used by the online credit marketplaces to optimize their loan repayment success by targeting the right borrowers and modifying their loan structure if required.
Presented and Published at: Proceedings at 5th International Conference on Business Analytics and Intelligence on December 2017 at IIM Bangalore