AI/ML Disruption- The New Normal
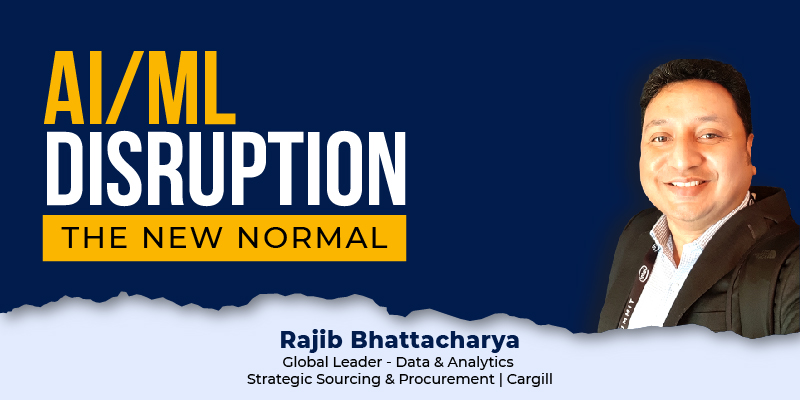
AI/ML Disruption- The New Normal
The emergence of the pandemic had created an impact not only on the global economy but also disrupted the technology at large. Likewise, AI/ML disruption is also creating breakthrough changes in multiple industries by simplifying lives and allowing businesses to work efficiently. AI/ML disruption has been predominantly creating changes for the last 5 years. AI and ML are used interchangeably, even though ML is the subset of artificial intelligence.
Around 3 years back, self-service analytics and business intelligence were on top of the technologies. According to Gartner’s report of 2018, self-service analytics and BI users will perform more analysis than data scientists by 2019. However, the scenario has been changed due to the emergence of the COVID-19 pandemic.
Popular AI/ML Use Cases – Disruption is on us
Disruptive technologies such as AI, deep learning, and machine learning have been adopted by a plethora of industries or enterprises around the world. These technologies have the potential to improve the quality of business operations in various sectors.
Let’s explore some of the successful business use cases that were positively impacted by the adoption of AI/ML technology.
Uber: Uber is the world’s largest taxi company that rents out cars for the public to travel from one destination to the other. But, Uber as a company doesn’t own any of these cars as it only helps in establishing a connection between the passenger and the driver of the car.
Airbnb: The largest online marketplace for rental accommodations that are available all over the world. Airbnb connects people around the globe who need of accommodations for their holidays or vacations with the rental accommodations in that local area.
Amazon/Alibaba: These companies are the largest retailers in the world without having their inventories to sell. Even though Amazon has some products of their own, yet it comes only around 2 to 3 % of their total retail goods. These retail companies have large warehouses to keep the inventories of their vendors. When an order is placed, the order is shipped to the destination by these retailers.
Facebook: This social media platform doesn’t own practically any of the content published, even businesses are selling their products through Facebook. Facebook also gives recommendations like Friends and business suggestions based on the search history or contact numbers saved in your phone.
All these businesses are just connecting the customers with the vendors without having products of their own. Actually, behind the scenes, AI/ML algorithms are connecting the dots between the customers and vendors. With the support of AI/ML algorithms, these industry leaders are personalizing the customer experience through customer segmentation, customer profiling, etc. Based on the search history and minimal purchase patterns of the customer, the AI/ML algorithms in these business models will provide product recommendations to the customers.
DIKW Pyramid and AI Ladder
How to climb up the AI ladder?
Data Information Knowledge Wisdom (DIKW) pyramid is a method used to represent the relationship of data, information, knowledge, and wisdom. Each step in the pyramid answers the questions of the previous data to add value to the data. As you move up the pyramid, you have to answer more questions to receive data insights. As you reach the top of the DIKW pyramid, the data is enriched with more meaning and context providing insights.
AI ladder is very similar to the DIKW pyramid. In the AI ladder, data and IA are at the bottom of the ladder. Then, the ladder moves upwards with analytics, ML, and finally to become an AI-enabled organization.
What is IA? IA is the abbreviation of Information Architecture, which consists of data warehouses, data lakes, and databases.
Using the data lake or data warehouse in the AI ladder, the data experts will support the company to travel the analytics journey. As ML cannot be applied to an unstructured dataset, the data expert has to transform it into structured data and apply analytics to the structured data. The analytics journey starts from descriptive analytics, diagnostic analytics, predictive analytics, to ML. Once machine learning is applied to the whole process, it will become an AI-enabled organization.
There is a popular saying in the industry that there is no AI without IA. This means that AI enablement is not possible without data. Some use cases that can be performed using unstructured data, but it comes only around 5% and the rest of the 95% should be done using structured data or IA.
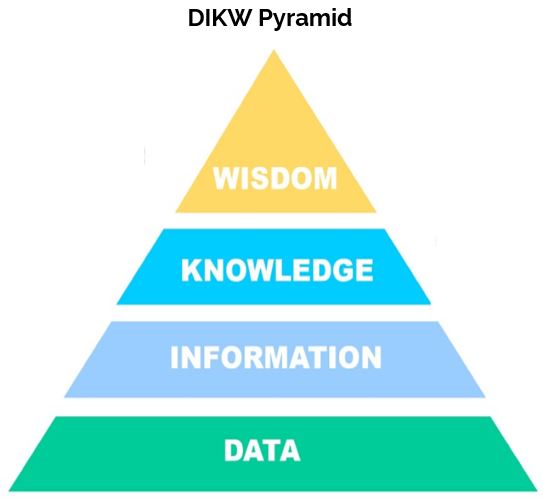
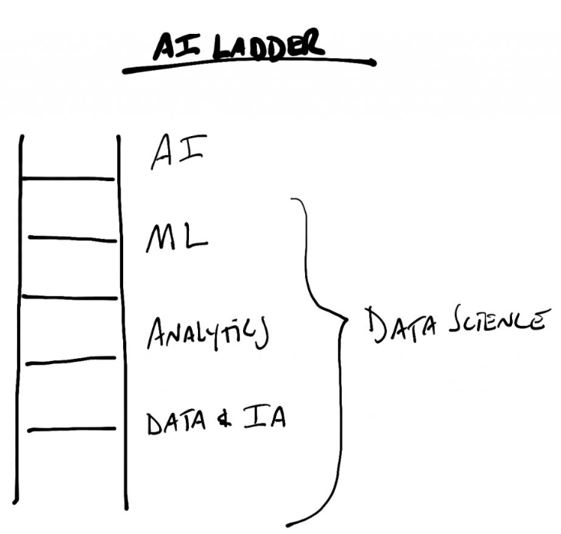
Branches of AI
The different branches of AI include machine learning, natural language processing (NLP), expert systems, speech, planning, and robotics. Even though AI/ML is used everywhere as a single term, in reality, AI and ML are different. To be precise, artificial intelligence stands taller than ML. Machine learning (ML) is only a branch of AI where you can find deep learning, supervised learning, unsupervised learning, NLP, and many more.
Artificial intelligence enhances the productivity of human beings or helps humans to do their jobs better. Hence, artificial intelligence is also known as augmented intelligence.
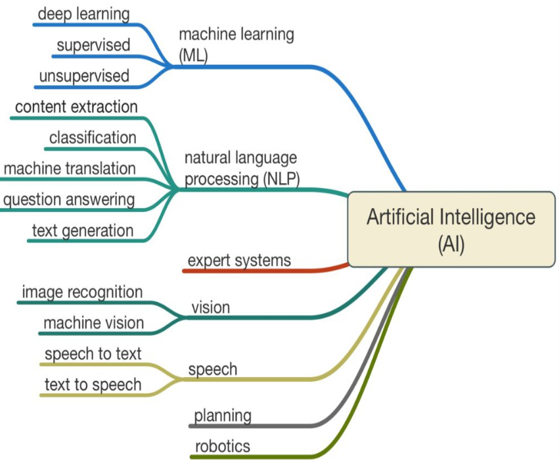
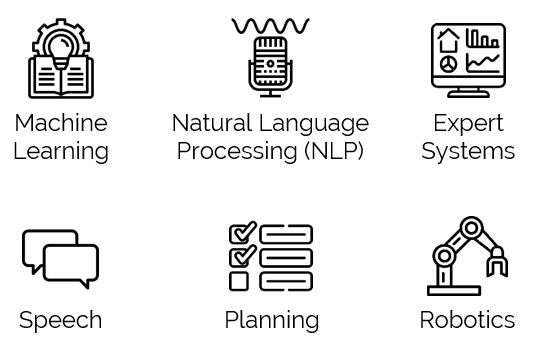
Data is a Natural Resource
“In God we trust. All others must bring data”- W. Edwards Deming.
If there is no data, it is almost impossible to climb the AI ladder. Listed here are some examples to understand the importance of data.
- Oil & Gas industry– In this industry, 80,000 sensors in a facility will produce 15 terabytes of data every day in an upstream or downstream operation.
- Public Safety– In New York City, 520 TBs of data are produced by traffic cameras daily.
- Energy & Utilities– Around 680+ million smart meters will generate 380 petabytes of data.
- Healthcare– In the healthcare industry, an equivalent of 300 million books of health-related data is produced in a lifetime of a human being.
By referring to these examples, you can understand that the world is sitting on top of a sea of data. However, the most difficult task is to create structured data from these data and perform the data analysis.
Data is everywhere
- Starting from a smartphone, tablet to laptop or PC, data is available in plenty and everywhere. Data can do amazing things such as enhancing one’s life, helping people, and propelling businesses.
- In today’s world, 80% of the data comes from prior information that is untapped or unstructured such as social media channels, emails, journals, blogs, sounds, videos, images, blogs, and feeds.
- 80% of the data present in the world are known as dark data as those data are unstructured data from where data experts can tap the information as it holds the most crucial insights required for faster and informed decisions
- The remaining 20% of data is the structured data that is available in data warehouses.
Data Analytics Challenges
AI required data to make the right business decisions. Today’s businesses are generating a great amount of data than before. But, what are the data analytics challenges businesses have to deal with to integrate AI?
Since businesses are generating more and more data on a day-to-day basis, they are facing challenges in data collection, data labeling, and data transparency. Without having quality data, AI models will not be able to generate accurate business insights.
Types of Analytics
There are four types of analytics such as descriptive analytics, diagnostic analytics, predictive analytics, and prescriptive analytics. Descriptive analytics analyzes what is happening in the business using comprehensive, accurate, and live data with effective visualization. Diagnostic analytics analyzes the data to answer or provide solutions to the questions as it uncovers the root cause of why it has happened.
Predictive analytics predicts what will happen in the future as it uses statistical algorithms, machine learning techniques and data to identify the probability of future outcomes. Prescriptive analytics is used to provide recommendations based on the data analyzed. Prescriptive analytics is the most useful of all the four types of analytics for businesses.
Concept of Cognition
Cognitive is an act of thinking, a mental process of understanding things through various thoughts and using shared or personal experiences. The evolution of AI has always been associated with the mimicking of human thoughts and actions. E.g. Human speech is mimicked in the form of text-to-speech and speech-to-text, which is known as speech synthesis. When it comes to vision, there are several computer vision use cases. Here, the AI/ML program will start thinking like a human and provides actionable insights.
AI is improving gradually to take up human tasks such as five human senses( hearing, smell, sight, touch, taste), extra-sensory perception, learning, remembering, language, empathy, social skills, motor skills, empathy, and problem-solving.
In a business scenario, a cognitive system must choose the best for the business to optimize the revenue.
Cognitive Systems
Cognitive systems are all about:
- Exploring data
- Finding new correlations
- Discovering details about existing correlations
- Finding new content in the data and providing new solutions
Cognitive systems will remove the bias, which is a human tendency. There are a lot of differences between cognitive systems and humans.
Humans excel in common sense, morals, imagination, compassion, abstraction, dilemmas, dreams, generalization, etc. However, cognitive systems excel in locating knowledge, pattern identification, machine learning, natural language, eliminate bias, and have endless capacity.
AI: Artificial or Augmented
What is augmented intelligence? Augmented intelligence is a cognitive technology developed to enhance the intelligence or expertise of human beings rather than replacing it. Hence, the focus is mainly on developing practical AI applications that support people to complete well-defined tasks, especially mundane tasks.
AI Use Cases in Telecommunication
- Churn Prediction– Predict potential churns and take corrective actions.
- Improves Customer Satisfaction– Chatbots for interaction, feedback collection.
- Combating Fraud– Finding trends of fraudulent activities and take corrective measures.
- Maintaining Standard of Services– Parsing millions of CDRs, finding bottlenecks, and provide immediate solutions.
AI Use Cases in Banking Sector
- Digital Assistants– Chatbots offer a great customer experience.
- Risk Management– Machine learning-based automated credit scoring models is helping the banking industry to process loans in a speedy manner.
- Fraud Management– Detecting fraudulent patterns and also offer a huge scope in cybersecurity issues.
AI Use Cases in Supply Chain
- Operational Procurement– Chatbots can streamline procurement related tasks.
- Supply Chain Planning– Machine Learning can help in forecasting inventory, demand, and supply for supply chain planning.
- Supplier Selection– Sourcing the right suppliers using machine learning and predictive analytics.
AI Use Cases in Retail
- Personalized Product Recommendations– AI-powered recommender engines provide recommendations based on analysis of thousands of data points.
- Next Best Offer– ML model targets potential cross-sell and upsell opportunities based on customer information and sales data.
- Customer Segmentation– AI solutions can automate the process of customer segmentation and provide better accuracy.
AI Use Cases in Insurance
- Customer Profiling – Unsupervised machine learning is used to segment customers with enriched profile data.
- Churn Prediction– Machine learning model targets potential churn cases based on customer information and take corrective actions.
- Lead Generation/Marketing Automation– Marketing automation tools with AI-powered solutions can sort, find and send the right information to the prospective customer at the right time to nurture the leads effectively.
Conclusion
Artificial intelligence/ Machine learning disruption enhances the resilience of organizations in these dynamic, unpredictable, and volatile marketplaces and optimizes growth performance in various industries. AI, disruption, and business growth continue to be the new normal and will stay here for long.