AI/ML in BFSI Use Cases and Careers
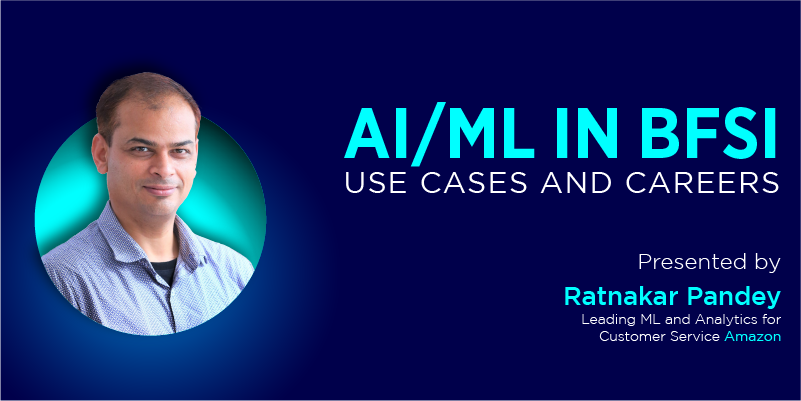
What is BFSI?
BFSI is an acronym for Banking, Financial Services and Insurance. BFSI sector consists of commercial banks, non-banking financial institutions, cooperative banks, internet banks, mutual funds, payment processing, insurance companies, pension fund, and other similar financial entities.
Classification of Banking Sector
The banking sector is classified into 3 major types of banking services such as Personal, Commercial and Central Banking. Personal banking deals with the banking services for retail customers (B2C) whereas commercial banking offers banking services for small and large enterprises (B2B). The central bank regulates the entire banking sector including commercial and non-banking financial institutions, e.g. Reserve Bank.
BFSI Products
An array of products/services are offered by the BFSI sector. Banking sector offers services such as deposits, savings, loan, credit cards, debit cards, checking accounts, demand drafts, etc. Financial services include payment processing, brokerage, stock, bond, Fintech, credit reporting, capital market, risk management, diversification, lending, investment advisory and so on. Insurance services include life insurance, home insurance, vehicle insurance, travel insurance, and many more.
How does the BFSI sector make money?
The revenue of the BFSI sector varies greatly on the services they provide. The main source of revenue in the banking sector is the difference between the interest rate earned from the borrower and interest rate paid to the depositor. Other revenue sources of banking sector include ATM fee, late fee, minimum balance fee, application fee, and commission through cross-selling.
In the insurance sector, the revenue is generated from insurance premiums, investment income like stocks and bonds, origination fee, third-party product commission, and other value-added services.
Other financial services generate revenue from asset management fee, payment processing fee, trading and brokerage, forex exchange, and other such services.
Where is Data Science used in BFSI?
A typical data scientist must answer all the questions as below:
- What has happened? – Descriptive Analytics including reporting
- Why it has happened? – Diagnostics Analytics like root cause analysis
- What may happen in future? – Predictive Analytics with ML Models
- What plan of action should be followed? – Prescriptive Analytics with recommendations.
How do data scientists answer these questions?
Descriptive analytics is used to analyze and interpret historical data to yield useful information to benchmark annual sales and revenues, which help the data scientists to explain what has happened. Dashboarding, visualization and various tools like Power BI, Python, tableau, etc., are used to describe the historical data in terms of statistics and numbers.
Diagnostic analytics is used as the second layer in which the data is analyzed to get valuable insights using facts and hypotheses, which will describe why it has happened.
By performing predictive analytics, one will be able to apply the learning based on the historical data to predict what may happen in future. In this stage, using appropriate AI/ML techniques predictive models are built
Finally, prescriptive analytics will decide what you should do to enhance the business outcome. Here the data scientist acts as a business consultant and solutionist. To figure out the possible actions for the next plan of action, it is imperative to consult with the business stakeholders at this stage.
Specific Models Used in BFSI
Specific business models are used in the BFSI sector and each model has its complexity depending on the business context. You may find more than 50 plus use cases used by the BFSI sector. In this section, only a few important models are discussed.
Customer Acquisition: Customer acquisition is about acquiring maximum customers with minimum cost. Identifying the most profitable customers, scoring them, designing campaign management to reach them through the right media channels, cross selling, customer response modeling etc are commonly used by all companies today and are applicable to BFSI segment as well. To achieve this, different types of machine learning models are used such as, campaign response modeling, market mix modeling, product/services optimization using A/B testing, fraud propensity modeling, and risk propensity modeling are examples.
Portfolio Management: In portfolio management, the focus area will be calculating customer lifetime value (CLTV) with the organization. The business models used to validate the customer value are customer lifetime valuation, customer segmentation, default likelihood modeling, product usage segmentation, and fraud prevention.
Deepen Engagement: Various machine Learning models are used to increase customer engagement including cross-sell and up-sell, product bundling, next best product, price and offer sensitivity, and improving customer satisfaction and net promoter score.
Customer Retention: Customer retention is measured by different ML models such as attrition propensity modeling, proactive retention campaign for high-value customers, discounting and product restructuring.
What is driving the need for data science in BFSI?
What are the driving factors for BFSI to tap on AI/ML? These models are evolving faster than ever. Why?
Some of the reasons are:
- Personalization of products and services
- Digitization and evolution of apps
- Real-time banking with anytime-anywhere banking
- Diminishing customer loyalty
- Stronger security regulations
- New Fintech entrants in the market
- Internet of Things (IoT) and new data streams
- Customer expectation to enjoy a consistent omni-channel experience
How big is the need for Data Scientist in BFSI?
Almost 41% of the jobs advertised for data science and analytics professionals are from the BFSI sector. According to NASSCOM, this sector in India is expected to increase by $16 billion by 2025.
What are the skills required to be successful in the field of AI/ML?
The skills required to be successful in AI/ML domain, especially in BFSI sector are:
- Love for numbers and quantitative stuff
- Good understanding of banking products
- Grit to keep on learning
- Coding skills (Python, R, SAS, SQL, Excel, and other)
- Presentation and story-telling skills
- Passion for solving problems
- Customer centricity
- Structured thinking approach
- Willingness to learn statistical approach
Wrapping Up
BFSI industry can make use of AI/ML capabilities for better customer experience and to enable 24/7 customer interactions. Artificial intelligence and machine learning applications are helpful to extract meaningful insights from the raw data sets. This insightful information can be used to solve complex data-driven problems in the BFSI sector.
References
Wikipedia: https://en.wikipedia.org/wiki/BFSI
For more details, refer RP’s blog: https://datafai.com/banking-and-financial-services/
AUTHORS
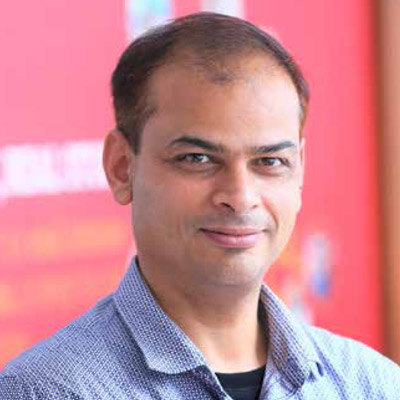
Ratnakar Pandey
Leading ML and Analytics for Customer Service Amazon