Auto-Detection of Click-Frauds Using Machine Learning
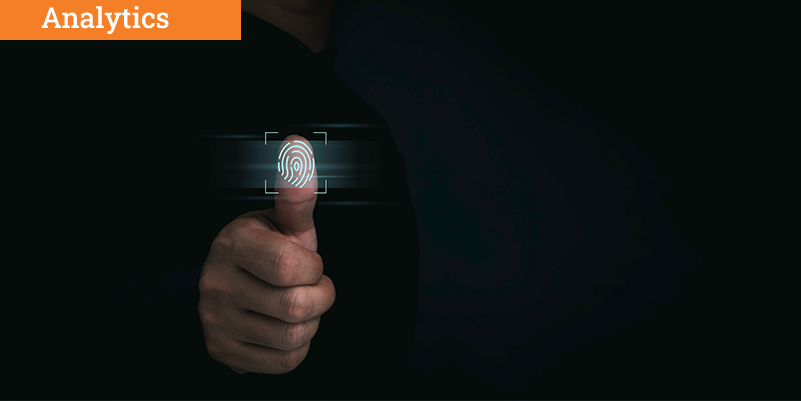
Abstract
Fraud is increasing the risk of online marketing, ads and e-business in existing web advertising activities. Online advertising has become one of the leading funding models for websites. Since large amounts of money are involved in online commercials, unfortunately, malicious parties try to gain unfair advantage.
Even if the online advertisers make permanent efforts to improve the traffic filtering techniques, they are still looking for the best protection methods to detect click frauds. Click – Fraud occurs by intentional clicking of online advertisements with no actual interest in the advertised product or service. Click fraud is major threat to advertisement world that affects the revenue and trust of the advertisers also. Clock fraud attacks are one instance of such malicious behaviour, where software imitates a human clicking on an advertisement link. Hence an effective fraud detection algorithm is essential for online advertising businesses.
The purpose of our paper is to identify the precision of one of the modern machine learning algorithm in order to detect the click fraud in online environment. In this paper, we have studied click patterns over a dataset that handles millions of click over a few days. The main goal was to assess the journey is a user’s click across their portfolio and flag the IP addresses who produce a lot clicks but never end up in installing the apps. We have focussed on the issue while using various single and ensemble-typed classification algorithms for the fraud detection task. As single classifiers, we employed the support vector machine and kNN algorithm. We have also used a decision tree based ensemble classifiers, which have been used in the data mining.
Published in:
7th International Conference on Business Analytics and Intelligence 5-7 December 2019 IIMB