Investigating Super Learner for Credit Risk Modeling In Mortgage Scenario
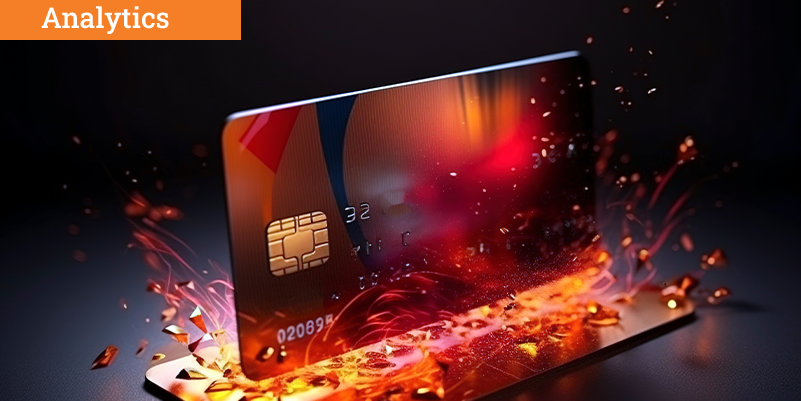
Abstract:
Customer lifetime value has emerged as an important metric for identifying and reaching out to Customers who make larger and more frequent contributions. As a result, this parameter is dependent on the marketing industry. It is critical to understand the value of a customer’s purchases and to recurrently monitor their transaction frequency and value to accurately determine their Customer Lifetime Value (CLV). Also, for any retail company, predicting sales is one of the most crucial business challenges. A company can better manage its inventory if it can forecast how much of each item it will sell each month. Additionally, sales forecasts assist in focusing marketing efforts to improve sales prospects. In order to implement the concept, a two-step strategy was taken. It is begun by estimating the frequency of future transactions from clients. The rate at which users will eventually leave the system has also been anticipated by us. Pareto/NBD or BG/NBD have been utilized to find them. These findings were utilized to determine the monetary value of our consumers. Additionally, the customers have been segmented based on RFM values, and then each group is examined separately in terms of Revenue with Frequency, Revenue with Recency, and Recency with Frequency. Furthermore, in order to forecast the monthly sales volume of each item, a machine learning algorithm has been developed. Quantity is therefore the target variable. Since it is a continuous variable, a regression algorithm will be used.
Conference Name: 9th International Conference on Business Analytics and Intelligence (IIMB – BAICONF2022)