Pattern Discovery and Forecasting of Attrition using Timeseries Analysis
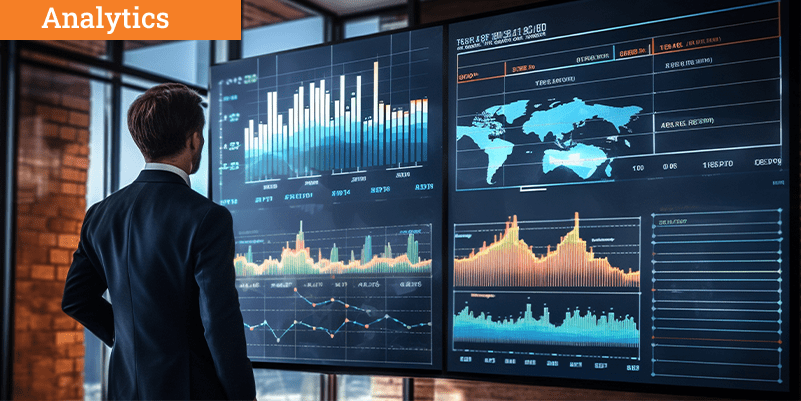
Abstract:
Attrition is the term used to describe when employees leave a company either voluntarily or involuntarily, for any cause including retirement, termination, death, or resignation. When employees leave, they take with them priceless tacit knowledge. Though it is a reality for any industry, if the rate of attrition is very high, it creates enormous pressure on the process to function effectively. This is precisely what a leading organization’s transportation Line of Business (LOB) is going through where attrition is hovering around 34% for the last three years. Time and again, it has struggled with managing a healthy attrition rate. As a result, there has been a constant occurrence of missed Service Level Agreements (SLAs) resulting in huge penalties.
For managers, managing workload has become extremely tedious in the current context. With the constant change in the management team, the focus keeps shifting from one approach to another.
Keeping the above problem in mind, this study aims to forecast attrition using time series analysis at various levels based on only the attrition data available for the last fourteen months. The hypothesis here is, if probable attrition is forecasted well in advance, a plan can be put in place to hire employees and make them available whenever there is demand from contract managers. This in turn can help individual contract managers manage their workload efficiently, and reduce their missed SLAs, thereby reducing penalties.
The proposed solution is to compare various Time Series Forecasting techniques like Auto-Regressive Integrated Moving Average (ARIMA), Seasonal Auto-Regressive Integrated Moving Average (SARIMA), Exponential Smoothing (ES), Holt-Winters (HW), Moving Average, Ratio to Moving Average, based on attrition date of the last 12 months. The forecasted data is then compared with the following two months’ attrition data to arrive at the best possible solution.
The novelty of this study is the use of time series forecasting techniques to forecast future attrition trends specifically based on attrition data, which has not been explored much. This forecasted data can be used to better workload management which in turn is expected to reduce missed SLAs and penalties.
Keywords: Forecasting, Timeseries, ARIMA, Seasonal ARIMA, Exponential Smoothing, Holt-Winters, Data Discovery, Pareto, Trend Analysis, Regression, Moving Average, LSTM
Journal Name: Lecture Notes of the Institute for Computer Sciences, Social Informatics and Telecommunications Engineering
AUTHORS
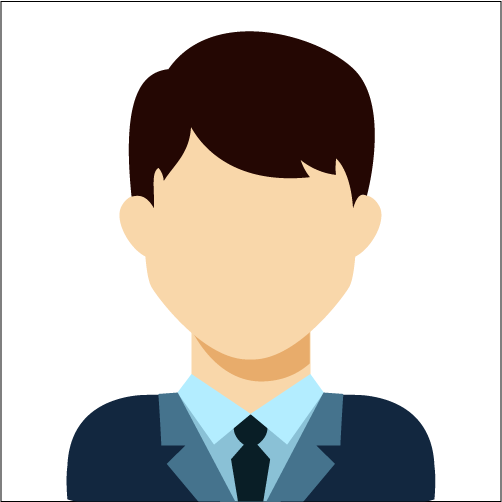
Saumyadip Sarkar
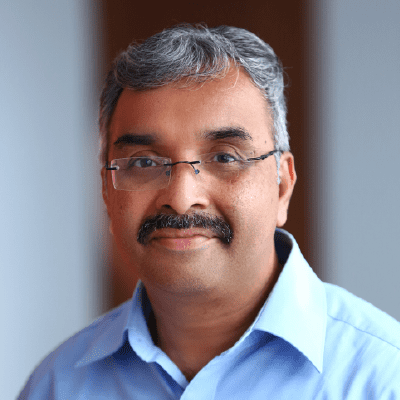