Virtual Market Survey on Automobile Infotainment Gadgets
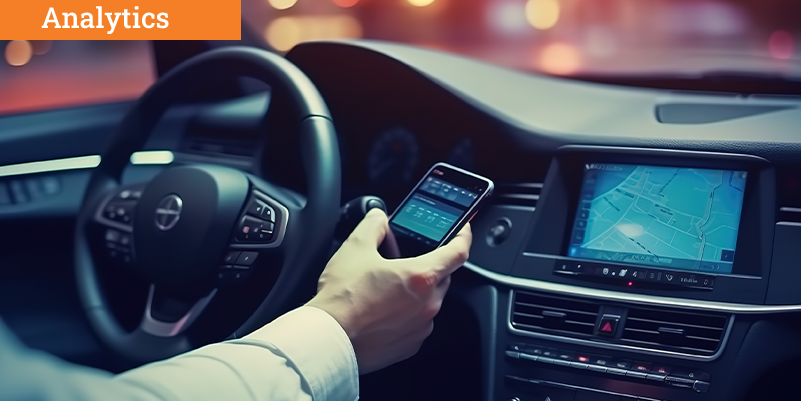
Abstract
When technology and socio-economic patterns evolve, so will our way of gathering feedback from customers. Today, it is easy to collect actual customer behavior data, to the point where analysis (or data mining) is much more challenging than data collection. Twitter is another great source of ready-to-use information that can be analyzed using text analysis. In the modern era, the virtual marketing survey is all about results. Because of the enormous amounts of data available on Twitter, marketing is becoming increasingly common for many companies as it has a direct connection to increasing revenue. Significant work was done in the fields of Automotive Gad gets and Twitter Analytics in (In-Vehicle Technology Survey[1],Twitter Sentiment Analysis[2], Twitter Brand Sentiment Analysis[3], Twitter Product Assessment Data [4], Product Review Data[5] and Consumer Insights[6]). However, the work done on Automobile Infotainment Gadgets Virtual Market Survey is limited. In this paper, they focus on the issue of defining Virtual Market Survey in Automobile Infotainment Gadgets using Artificial Intelligence and Machine Learning methods to collect Automobile Infotainment Public Opinion Tweets. Publicly available tweets provide useful and in-depth insights into the real-time virtual market survey of automotive infotainment gadgets and provide motivation through online influence to others. We collected data from the desired source (here, Twitter) to perform a virtual market survey. The Tweets are collected for the desired time period of analysis using the streaming API of Twitter. The obtained text format is translated according to convenience. With standard Text Analysis, this information undergoes various steps of pre-processing. The data is tokenized and cleaned before analysis. Before analyzing, the data is tokenized and cleaned. The cleaned text data is used for data exploration. The classification models are based on SVC, Decision tree, Random Forest, Gradient boosting and Gaussian Naïve Bayes. After the creation of the models, we work to train and valid ate the performance of the model using Confusion Matrix, Precision, Recall, F1-Score and Receiver Operating Characteristic Curve for each Supervised Classification Technique as evaluation metrics to understand the Classifier performance. Once the future trend of the infotainment gadgets has been identified based on the real-time virtual market survey conducted, the results of this virtual market survey could have positive impact on the automotive Industry.
Published in:
Proceedings of the Seventh International Conference on Business Analytics and Intelligence, December 2019, IISc, India.