CRM-Based Lead Scoring with Machine Learning
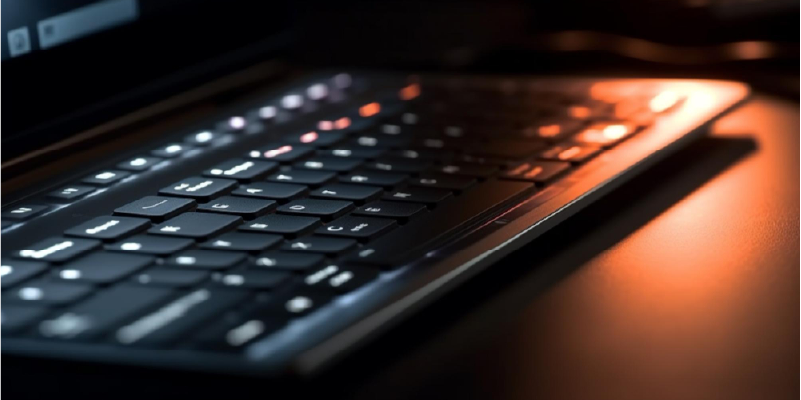
Abstract:
Betutelage is a startup organization that offers educational courses and provides live classes to audiences of different skill levels. They are a million-rupee revenue generator backed by investors who were impressed with their concept of providing valuable insights to students in areas where they can improve their focus on studies etc.
Betutelage needs assistance in predicting their leads. These leads represent their highest-paying enquiry conversion customers. Betutelage needs a model that can assign a score to each lead so that their customers will have a good conversion rate when the lead score is high and vice versa. By using this model, Betutelage aims to invest more time in non-converting leads and convert them into paying customers. They will also be able to reduce the cost of their campaigning in areas where there are no leads.
This paper has opted to study 4 classification machine learning models, which are Random Forest, Gradient Boosting, LightBGM, and Catboost, using CRISP-DM methodology with the data provided by Betutelage. The aim is to find the best model among these models that have the highest accuracy to convert leads based on both test and train data.
The outcome of this investigation demonstrates that Random Forest, with train and test accuracy of 94.3% and 92.02%, respectively, has the highest accuracy.
Keywords: Artificial Intelligence, Machine Learning, Deep Learning, Classification Models, Leads, Random Forest, Gradient Boosting, LightBGM, Catboost
AUTHORS
Rashmi Agarwal
Associate Professor