Predicting Customer Lifetime Value using ML: A Comparative Analysis !
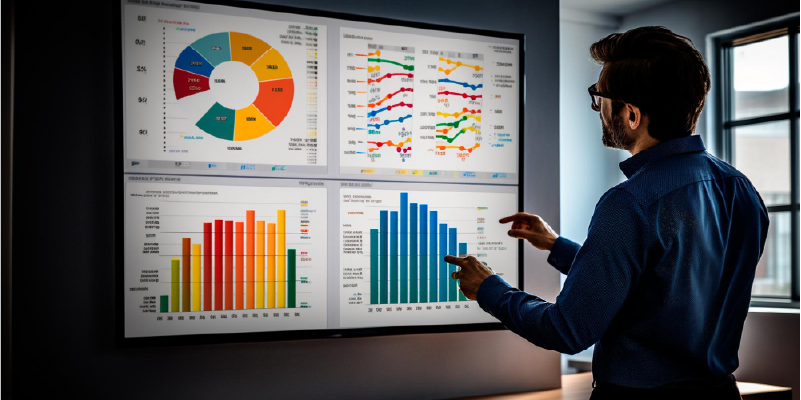
Abstract:
For businesses to at least sustain in competitive markets, the key is to identify such categories of clients and target only the clients that add more value.
Brand awareness, client attitudes, or even sales and shares that fall under the marketing metrics category are not good enough to show a return on the investment in marketing the offering as these are traditional in nature. This drives the need to predict the Customer LifeTime Value (CLTV) for a business.
Financial metrics like the aggregate profit of a business or stock price which are measured, are useful with diagnostic capability. Recent studies have come up with the observations that there will be no uniformity across all clients being profitable. It may be a wise strategy to reach out to target some clients or allocate resources differently to specific segments of clients.
CLTV is a key metric that can be predicted for a customer to know the future value that the customer can add to a business or firm. The value is realized in terms of the profit a firm can make from all the purchases a customer makes with the firm. Additionally, it signifies the long-term relationship the customer has with a firm.
As part of this study, CLTV is predicted using various linear and non-linear machine learning techniques. Predicted CLTV is being compared based on the key KPIs to identify the best technique that could be used. Based on the CLTVs, customers can be categorized into different groups like most profitable to least profitable. This categorization helps the marketing teams to come up with strategies that enable the business to tap the full profit potential of the customers.
Success of a firm depends on the firm’s ability to attract and retain customers that are loyal and valued to the business.
Keywords: CLTV, CART, CHAID, ExCHAID, MLP, RBF, KNN, QUEST
AUTHORS
Dr. Rashmi Agarwal
Associate Professor