An Improved Method PhishingURL Detection using Machine- Learning
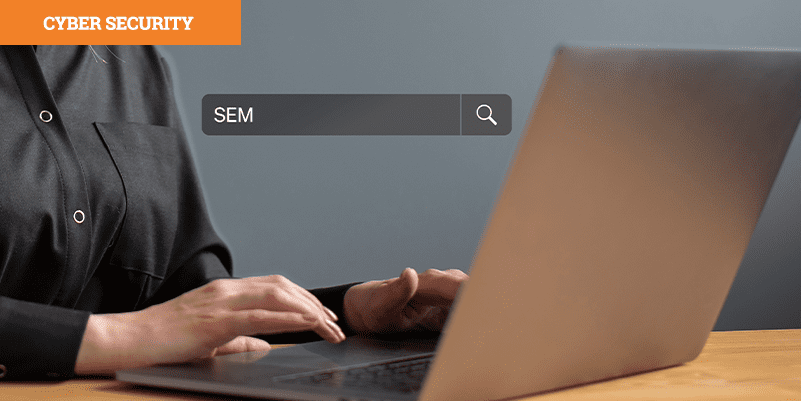
Abstract
The Internet has become an integral part of our lives over the past few years, providing us with essential services ranging from online banking to communication and travel. However, the increasing dependency on the web has also led to a surge in cyber-attacks and fraudulent activities, making it crucial to identify malicious websites and protect ourselves from them. Phishing attacks are the leading cause of internet data breaches. According to the FBI, these attacks are expected to increase each year. Shock-ingly, only 57% of organizations have URL protection in place. Successful phishing attempts can result in data loss (60%), system compromise (50%), and ransomware (47%). Phishing attacks target financial companies, social media firms, Software as a service company, and retail sellers the most. One of the most critical factors in deter-mining whether a website is safe or not is its Uniform Resource Locator. Despite nu-merous measures taken by cybersecurity experts to identify phishing URLs, attackers always find new ways to attack and breach existing anti-phishing defenses, causing harm to innocent internet users. To combat this growing threat, an improved approach to detecting phishing URLs has been proposed. A dataset consisting of both normal and malicious URLs was used, and five supervised machine-learning algorithms were ap-plied to it. Feature engineering was performed, and 14 essential attributes contributing to a phishing URL were extracted. To test the URLs, a DNS toolkit called DNSpython, which queries and resolves name servers, was used, and the DNS records of the URLs were used as the target variable. The toolkit was specifically selected due to its ability to effectively query and resolve DNS records. The DNS records of the URLs were as they provide essential information about the IP address and domain name associated with the URL. After experimenting with the dataset, it was concluded that the Random Forest algorithm provided the highest accuracy score of 95.38% among all models. This algorithm proved to be the most effective in detecting phishing URLs and yielded better results when compared to other models. Additionally, a web interface was built with the attributes from the RF classifier to show the prediction of the URLs based on the detection, providing a user-friendly and efficient way to identify malicious websites.
Presented at:
Eigth International Conference on Smart Trends in computing and communications