Transfering Learning for Speaker Verification with Short Duration Audio
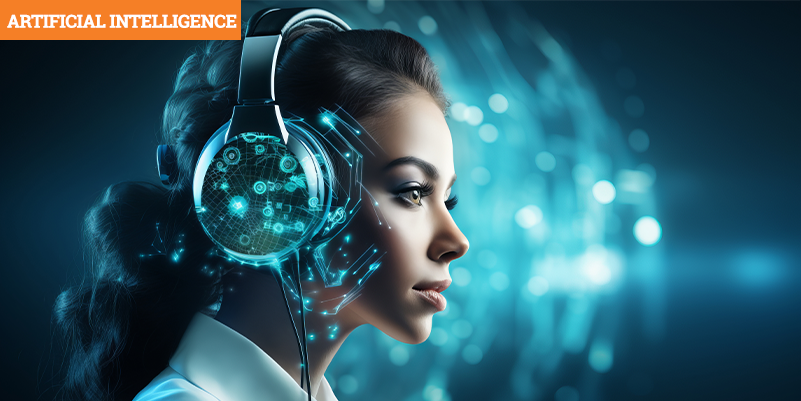
Abstract: Speaker verification or identifying the legitimacy of a speaker’s iden-tification from their voice, is a fundamental problem in speech processing and biometrics. The growing requirement for efficient and secure speaker verification systems, particularly in applications such as voice assistants, authentication, and communications, has increased the necessity for reliable verification even with short-duration audio samples. Short-duration audio provides a considerable bar-rier because of the limited information available for processing. In this research, it is investigated to make use of the transfer learning strategies to meet this diffi-culty and improve speaker verification systems’ performance. The primary goal of this research is to investigate how transfer learning can be used to improve speaker verification while working with short-duration audio. To get started, a varied dataset of brief audio samples from a variety of speakers, languages, and settings is gathered. To improve the quality and diversity of the dataset, these audio samples are then put through preprocessing procedures such as noise re-duction, feature extraction, and data augmentation. To validate speakers based on data samples collected from a few speakers, during this process, speech signals from each speaker are collected for roughly 20-30 seconds. The speakers are en-rolled using a pre-trained 3D Convolutional Neural Network (3D-CNN) at the enrollment step. Speaker Verification is performed on the data samples by enrol-ling the necessary speakers and creating a speaker model for each speaker. The speakers are then validated at the authentication stage.
Prsented at: Eigth International Conference on Smart Trends in computing and communications