Building Predictive Models for email campaigns
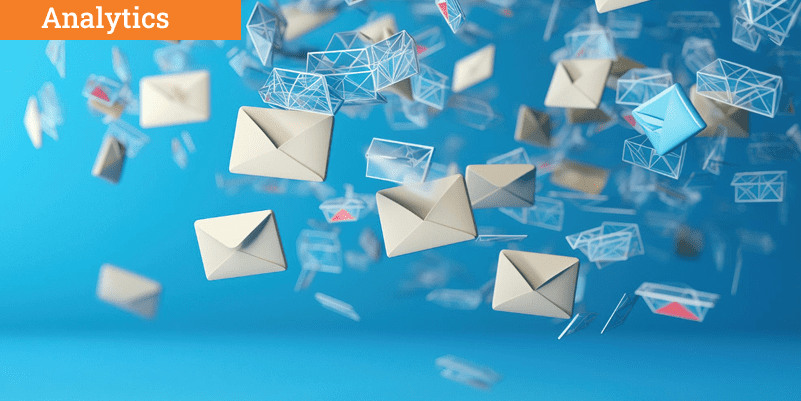
Abstract
Email marketing is the extraordinarily powerful virtual marketing approach of sending emails to prospects and potential customers. Effective e-mail marketing converts possibilities into customers and turns one-time buyers into dependable, raving lovers. Despite the rise of social media and unsolicited spam, email still remains the most effective way to nurture the customers. Companies are spending a lot of time in writing that perfect email, laboring over each word, innovative subject lines, senders name and catchy layouts on multiple devices to get them best in-industry click rates & view rates.
Objective of this study is to analyze if we can optimize our email marketing campaigns using different analytical techniques for predicting the click probability of email campaigns. For the purpose of our study, we have used the data sets from the “Lord of the Machines” Data Science hackathon from Analytics Vidhya. The datasets used in this hackathon contained real-life cases, where more than 1 million emails/newsletters sent by Analytics Vidhya for promotional and campaigning purposes were included. The data was shared after masking/removing some personal and sensitive information.
This enabled us to work on a real-life problem, currently being encountered by the email marketing teams in various domains. The solution was to come up with a predictive model that lets us classify between the users based on their click probabilities and thereby, selecting the right segment of customers for the different types of campaigns. To come up with the best solution for this challenge we have used various supervised machine learning algorithms such as Naïve Bayes, Logit, SVM, Random Forest etc. (which also involved a bit of text mining).
Published in: Proceedings of the Sixth International Conference on Business Analytics and Intelligence, December 2018, IISc, India.