Convolutional Neural Network Model for Detection of Diabetic Retinopathy in Clinical Practice
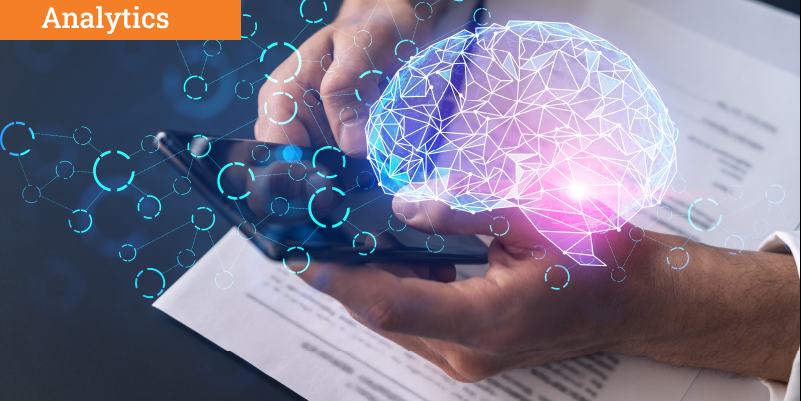
Abstract
As per the estimates are done in 2010, diabetic retinopathy accounts for approximately 2.6% of blindness worldwide. The microvascular changes due to prolonged diabetes result in several changes in the retina- such as microaneurysms, haemorrhages, hard exudates and cotton wool spots that characterize different stages of diabetic retinopathy. Visual impairment may get noticed by the patient very late in the disease progression- at a stage when preventive measures might have very limited benefit. Early detection can serve as a warning and can facilitate adopting preventive measures to slow down the progression of the visual impairment due to diabetic retinopathy. Control of blood sugar (glycemic) and blood pressure are major factors that can slow down the progression of diabetic retinopathy.
Automatic detection of diabetic retinopathy can be very helpful, especially in the field (medical camps) where adequate trained staff might not be available, or when the volumes of people to be screened are so large, that it is physically challenging for a handful of trained medical personnel to detect the condition manually. Automatic detection of diabetic retinopathy may be done by numerous techniques like mathematical morphology, artificial neural networks, pattern recognition, region growing techniques, fuzzy C-means clustering and Gabor filter banks.
We have developed a convolutional neural network model to detect diabetic retinopathy. In this model, we have used Kaggle dataset. The high-quality retinal images from this dataset have been used to train and test the convolutional neural network model to detect diabetic retinopathy by identifying microaneurysms, haemorrhages, hard exudates and cotton wool spots.
Presented and Published in: Proceedings of the Sixth International Conference on Business Analytics and Intelligence, December 2018, IISc, India.