Deep Learning and Fraud Detection in Credit Card Transactions
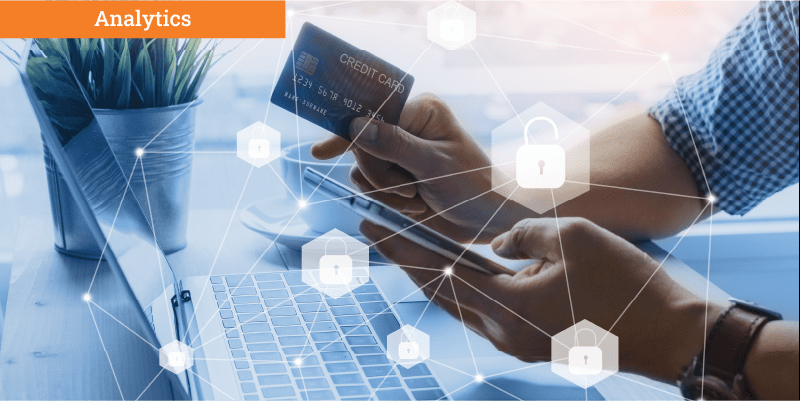
Abstract
Per a recent article from CNBC News, around 15.4 Million American Consumers were victims of identity theft or some other fraudulent activities in 2016, resulting in the almost $16 Billion losses to the affected consumer. Needless to say that real-time fraud detection has become an imperative for the global banking and financial services industry to survive in a very competitive and fast-changing environment. Currently, most of the players in the BFSI industry use Machine learning techniques such as logistic regression, decision tree, Elastic Net, Gradient Boosting Tree to identifying potential fraud cases. However, with ever-evolving modus operandi of fraudsters, the banks need to deploy self-learning/ deep learning algorithms such as Autoencoder, LSTM and other Deep Learning Neural Network to stay a step ahead of fraudsters. In this paper, the authors have built an algorithm using a combination of two different deep learning networks- Multilayer Perceptron and Autoencoders to classify the labelled fraud cases of a European Credit Card company to achieve the highest levels of precision and recall.
The dataset was split into train and test data. The experiments was conducted by varying the hyper-parameters of the algorithm such as the number of layers, number of neurons, drop-out rate, learning rate etc. using grid search. Two metrics, precision and recall were used to compare different settings and identify the best hyperparameters of both networks. More than 80% of the precision and recall values attained during the trials reinforces the belief the deep learning network are ideal candidates for real-time fraud detection on large, multimodal and heterogeneous data prevalent in the BFSI industry.
Presented and Published at: Proceedings at 5th International Conference on Business Analytics and Intelligence on December 2017 at IIM Bangalore