Prediction of Fatal Accidents in the Petrochemical Industry with Machine Learning
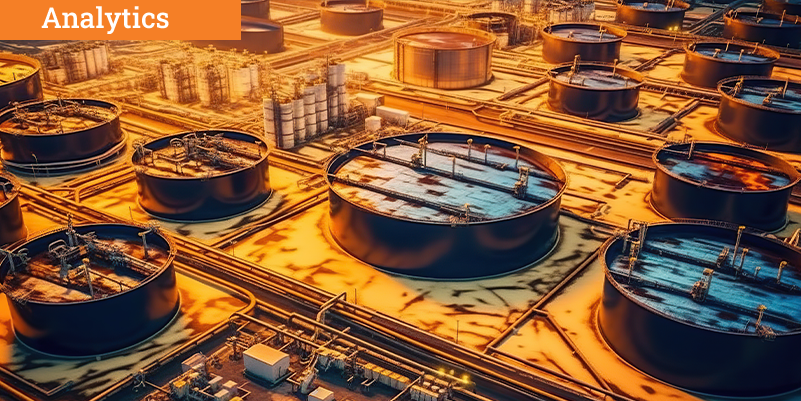
Abstract
There is an urgent need for energy companies to understand why employees still suffer injuries/accidents. The full potential of historical incident data is mainly un-explored and unexpressed. This study aims to question some preconceptions and identify opportunities for the future by better understanding the causes of catastrophic events, their effects, and the lessons that can be learned from them. In this paper, structured and unstructured data are taken to predict fatality. Structured data is analyzed with machine learning models and a combination of structured and unstructured data is analyzed with machine learning and deep learning models. For the structured data, out of all models, XGBoost gives an accuracy of 81%. And, for the combination of structured and unstructured data, Naïve Bayes with TF-IDF vectorizer gives an accuracy of 92%. Hence, this paper concludes that the Naïve Bayes with TF-IDF can be considered to predict the fatality of accidents with reasonable accuracy for a combination of structured and unstructured data.
Keywords: Occupational Accident, Naïve Bayes, XGBoost, Classification, Decision Tree, Logistic Regression, Deep Learning (DL), BERT, SVM.
Conference Published in: 4th international conference on recent trends in communication and intelligent systems (ICRTCI 2023)