Response Modelling using Support Vector Machines & Naïve Bayes
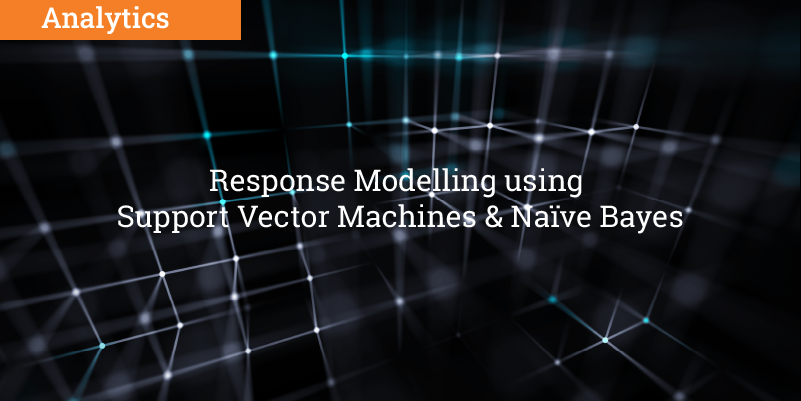
Abstract
Predictive analytics has become the crucial differentiating factor for highly competitive online retail companies in creating optimised solutions to provide the “right offer, right person, right time” to their customers. Response modelling is one of the important predictive modelling techniques used to get insights into the responses or behaviour of the events like repeat purchase by customers. It uses data mining techniques to find similarities between respondents from previous historical data to predict who is likely or not likely to respond in the near future.
Our experiment involves supervised learning methods, application of a Support Vector Machine (SVM) and Naïve Bayes are used to evaluate the data set for repeat purchase behaviour for an e-commerce business. We use Naïve Bayes as a baseline model and further we extend our work to more advanced classifier technique like SVM to evaluate the performance accuracy. The support vector machine is called as a linear classifier which creates a hyperplane that separates positive samples from negative samples.
The datasets used for this paper contains transaction data for an online retail shop. The customer level RFM (Recency, Frequency and Monetary) data is extracted for analysis. This paper also provides various evaluation measures for response models in terms of predictive accuracy, computational efficiency, and mid-classification error. The results of the experiments will be discussed in this paper enabling the retailer to deploy the right models with superior performance.
Presented and Published at: Proceedings at 5th International Conference on Business Analytics and Intelligence on December 2017 at IIM Bangalore