Predicting the Likeliest Customers; Minimizing Losses on Product Trials using Business Analytics
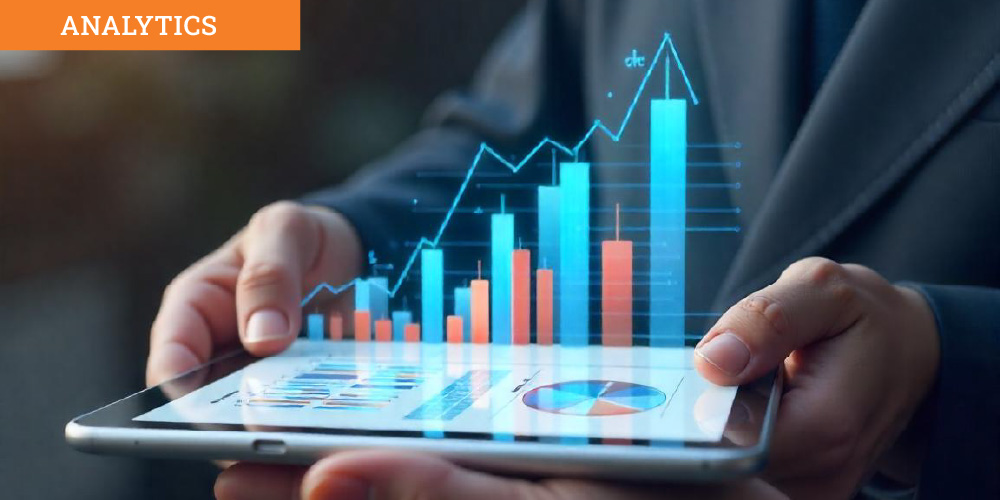
Abstract: A product trial is a great way of marketing. It offers a first-hand experience that a customer can use to evaluate the product and decide whether to buy it. However, there are expenses involved in every trial. If the buyer decides not to purchase the product after the trial, this expense gets wasted. The lower the conversion rate, the higher the loss that the company must face. To reduce these losses, a strategy should be developed on which studies should be conducted. One such business case is the subject of this paper. A software development company has launched a new product and given its current customers access to the product’s trial version. A loss of $8.5M resulted from 56% of trial customers not buying the product. The business is therefore looking for strategies to reduce this loss. This paper aims to present the work which is done to solve this business problem. The goal is accomplished by employing analytical methods to determine the most likely clients. The data provided by the company is used to build binary classification models. Another way to find the likeliest customer is based on the purchase pattern of the customers. Affinity analysis is done on the data to identify the set of products with which the new product is frequently sold to target the customers who have purchased those products for the sale of the new product. Among the binary classification models that were built, the Random Forest model outperformed other models with an accuracy of 83%. This enables the business to take calculated decisions while extending the trial to the customers. Alternatively, Market Basket Analysis, with an accuracy of 88%, discovered a set of two products, the existing buyers of which are more likely to buy the newly launched product. This information not only helped find the right customers but also paved the path for cross-selling of the new product.
Published in: Lecture Notes of the Institute for Computer Sciences, Social Informatics and Telecommunications Engineering
Presented in: EAI ICISML 2022 – EAI International Conference on Intelligent Systems and Machine Learning