Anomaly Prediction in Real-Time Water Flow Data-Machine Learning Vs Statistical Models
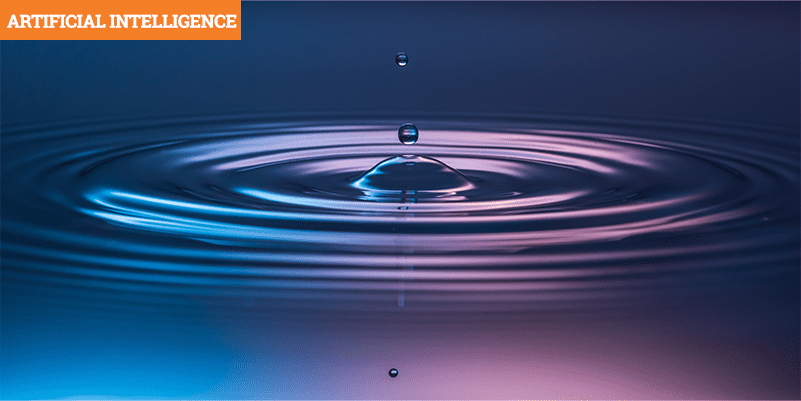
Abstract
Industries are at the vanguard of the looming catastrophe in a time of growing worries about resource depletion and water scarcity. Additionally, the enor-mous financial outlays needed to secure water sources and treatment methods highlight the underlying cost of water. Industries must optimize their water consumption to re-duce this mounting financial burden and the negative environmental effects of exces-sive water use. This study is highly significant since it takes a novel technique to predict anomalies in industrial water use. This research paper focuses on a thorough compara-tive study to assess two popular approaches for anomaly prediction in real-time water flow data: Statistical Models and Machine Learning Models. This research makes use of dynamic machine learning models as opposed to conventional techniques, which concentrate on establishing a single static threshold value. These models adjust to the constantly shifting circumstances in industrial operations. Water usage can vary signif-icantly in dynamic industrial activities, such as those in the Food and Beverage (F&B) sector, depending on output levels, seasonal conditions, or even unplanned equipment failures. As a result, choosing a single threshold number is frequently insufficient since it ignores the subtle patterns and trends in the data. The dataset being looked at comes from an electromagnetic water flow meter that has an Internet of Things (IoT) device attached, allowing for easy data transmission to the cloud. With the help of a high-quality dataset effectiveness of several models is investigated, including the well-known statistical approaches with Weighted Moving Average (WMA), Simple Moving Average (SMA), Exponential Weighted Moving Average (EWMA), Auto-Regressive Integrated Moving Average (ARIMA), that are known for their skill in identifying time series anomalies. Parallel to this, we make use of machine learning models like K-Nearest Neighbors (KNN), Isolation Forest (IF), Support Vector Machine (SVM), and Gaussian Mixture Model (GMM) to make predictions. With an outstanding recall of 0.89 and a precision of 0.75, KNN performs admirably with an F1 score of 0.82.
Published in:Eigth International Conference on Smart Trends in computing and communications