Affordable ML Based Collaborative Approach for Baby Monitoring
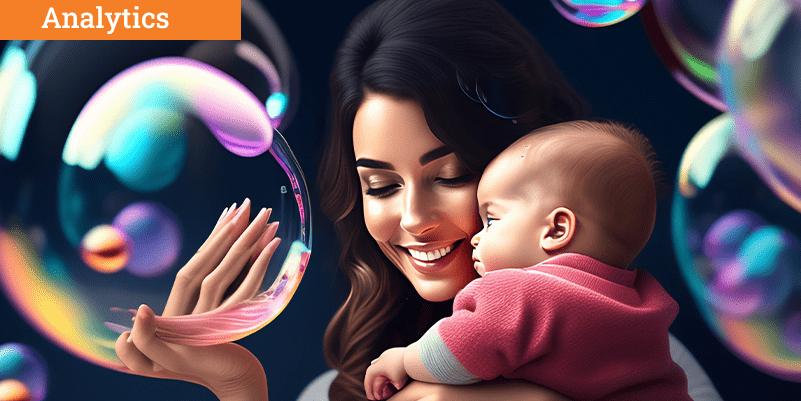
Abstract
There are numerous baby-monitoring devices available in the market that parents use to keep an eye on babies while they are away. The majority of them are reliant on the installation of expensive hardware, which many parents cannot afford. Another issue with these devices is that they detect high-pitched sounds and frequently give false alarms, causing both children and parents to be disturbed. The majority of smartphone applications in the market work on sound wave and only sound an alarm when the infant start crying. In this project, we proposed the design of a mobile application to detect the status of a baby inside a crib/ on a bed. The application will alert parents when their child requires assistance, will be able to determine whether the child is sleeping in a safe or hazardous position, and will keep track of the child’s sleeping patterns. It is less reliant on hardware, making it less expensive. Here the only requirement is two paired mobile phones with the application installed instead of expensivehardware (IoT-based devices). The application is utilizing the transfer-learning technique on tensor flow lite Mobilenet classification and SSD_mobilenet_V1_coco object detection models.The accuracy of the model is 97% for the Mobilenet classification model and 98% for the object detection model.
Published in:
International Journal of Research in Engineering and Science (IJRES- August 2021 Volume 9-Issue 8).