Credit Card Segmentation and Recommendation System
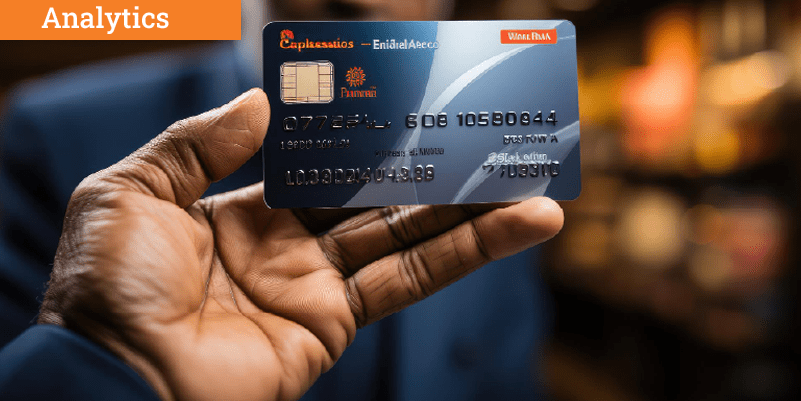
Abstract:
Credit cards are an integral part of the growing economy in the Banking and Financial industry. Credit card utilization adds a prominent value to the banks. Scraping the credit card data will help in identifying interesting patterns and characteristics among different features of the cards that in the future can be used by the banking and financial services to further increase their strength by credit card issuance and acquiring new users.
For a user, it is difficult to acquire the best card to fulfill most of their needs from the vast availability of credit cards. Thus, recommending a card that suits the customer’s needs is important. This further helps banks to identify and analyze different demographic characteristics and payment methods of customers. It also helps to identify potential customers and to implement target marketing.
This study is driven to develop and identify the credit card offers and group them into clusters for recommending a better card that serves the need of the customer. The data is collected from Banks by scraping their websites. There are 189 credit cards that contain 65 variables. The most important techniques used are Selenium Web driver, Beautiful Soup, Principal Component Analysis (PCA), K-means, and K-Nearest Neighbor (KNN).
Results indicated how credit cards are grouped based on how different offers and benefits they provide. Moreover, results revealed there are many offers available from banks but not every offer is necessary for analysis and grouping. Further, KNN is applied to identify the closest similar card to provide the credit card recommendation.
Keywords: Credit card, web scraping, clustering, recommendation, target marketing
AUTHORS
Ratnakar Pandey
Advisor and Consultant for Generative AI, NuWare
Shinu Abhi
Professor and Director - Corporate Training