Prediction of Delays in Invoice Payments using Machine Learning
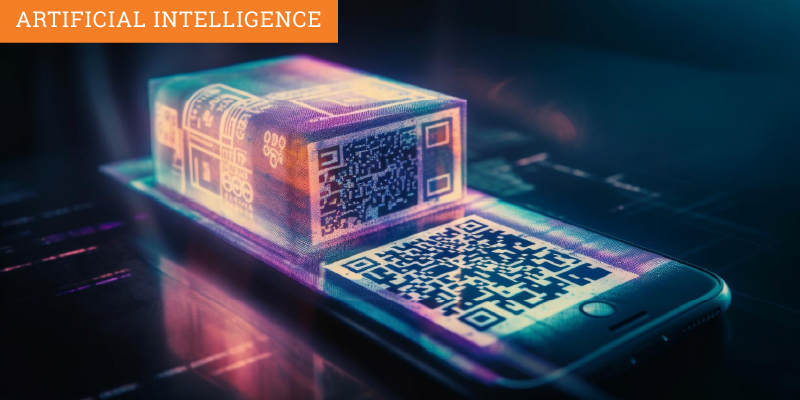
Abstract:
Accounts Receivable (AR), is the most valuable asset of an organization. If it is not managed effectively, it can cause the firm serious financial hardships. To gain an understanding of AR, it is necessary to recognize data patterns in order to forecast whether an invoice will be paid on time or will experience a delay.
It is extremely important for large organizations that deal with thousands of vendors to fulfill their service level agreements with them to avoid penalties. The data is collected from a multinational organization consisting of the past two year’s transactions. The research has been conducted by reviewing numerous papers related to invoice processing and different methodologies used to estimate consumer payment in AR.
The purpose of this paper is to present a supervised modeling solution for predicting the payment outcomes of newly created invoices, thus enabling collection actions tailored for each invoice or customer. Since this is a classification problem, an ensemble method of Random Forest and Extreme Gradient Boosting algorithms has been applied and has achieved the highest accuracy if an invoice will be paid on time or not and can provide estimates of the magnitude of the delay which can improve the prioritization of customers and facilitates the daily work of collection personnel.
It is estimated that the adoption of the model to prioritize the work of the Collection Management team will result in a substantial amount of savings. Consequently, the team would contact respective customers or account holders during the period in which the invoices are open, thereby reducing the organization’s accounts receivable.
Keywords: Machine Learning, Invoice Processing, Invoice Payment, Delayed Invoices, Accounts Receivable, Predictive Modeling, Feature Engineering.
AUTHORS
Rashmi Agarwal
Associate Professor