Optimizing the Manpower Planning with better utilization in E-commerce
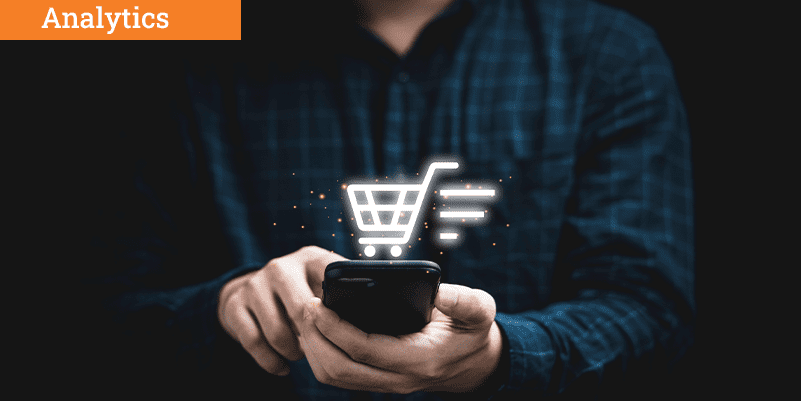
Abstract
Accurate forecasting and manpower planning as per the demand is one of the critical success factors of any e-commerce businesses. In a typical e-commerce business, to meet call demand without understaffing or over-staffing, we need to deploy robust techniques which are analytics-driven. A contact centre needs to come up with a solution, which can precisely predict how many agents are needed to handle the tickets. Proper forecast leads to proper staffing, meeting the service level, better cost management and hence, better customer experience. Current methods hardly can solve this problem since they either focus on short-term forecasting for the next day, week, or ignore call-holding time for call traffic prediction, hence this paper is to introduce analytically driven forecasting models with better accuracy and simultaneously provide the manpower required to be staffed. The sample data used for this research papers is taken from a retail e-commerce company with an in-house contact centre (customer support) in three locations (Pune, Bangalore & Hyderabad) with more than 1500 employees. Customer contacts the centre via calls/email/chats for any query/request/complaints. The company receives approximately 40000 tickets from these three channels. Currently the forecasting is done on excel based on the number of orders planned for the month against tickets received from customers. This leads to inconsistency in hiring manpower posing a risk of either less utilization or added pressure on the employees. So, this is one of the key challenges the companies face in the forecasting of calls/chats/emails in an hourly/daily/monthly basis as it tends to give higher deviation in the forecast, the accuracy level drops during the seasonal cycles (holidays, special events, promotional activities, etc.). Applying forecasting brings in many challenges like erratic business growth, seasonal factors, promotions/marketing events, unusual trends in purchases and tech-related issues which lead to incorrect forecasting. Different Time Series models (ARIMA, Holt-Winters, and Linear Regression along with Simple moving average) have been investigated to achieve the best prediction or accuracy, and which is dynamic in nature. The proposed forecasting model will be deployed in production using web application. This will give the output of the model providing the best accuracy, which is taken as an output to predict the no. of agents required to be staffed for the day. The application will predict the calls/chats/emails for a given date (with peak and nonpeak time) and provides the concurrent logins required with 75%- 80% utilization. This will also help in moving the employees from one queue to another queue for better utilization. With this type of approach, call centre workforce management (WFM) can arrange staffing availability efficiently based on volume predictions, hence optimizing the manpower and reducing the cost to company by around 10%-20%. The results suggest that call centre managers should invest in the use of forecast models which describe dynamically changing volume and utilizing the agents in better productivity.
Published in:
Proceedings of the Seventh International Conference on Business Analytics and Intelligence, December 2019, IISc, India.