Future of Data Engineering
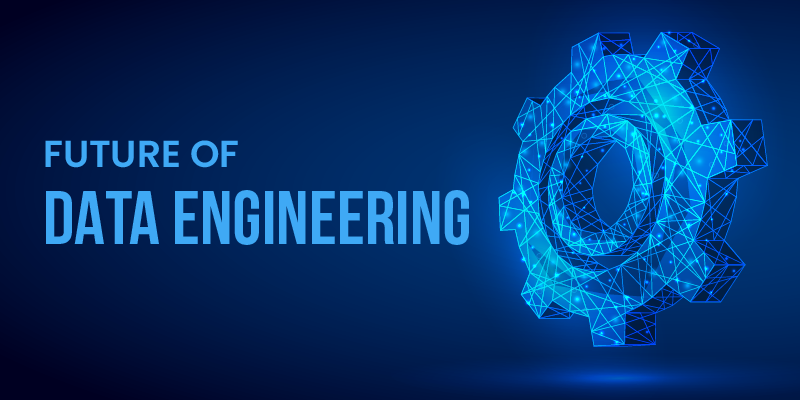
Data is ubiquitous today and is becoming the key differentiator in every industry or sector. All these exponentially increasing data, need to be extracted and analyzed for making data-driven business decisions. Thanks to big data technologies, today organizations can harness the power of enterprise-wide data.
The majority of organizational data consists of sensitive information and innovative ways have to be used to manage real-time data requirements. The demand for data professionals is increasing as never before. According to Forbes, data scientists, big data engineers, and machine learning engineers are in high demand as per this article.
Several organizations are redefining their business goals based on the analysis of data. As the data transforms businesses with ever-evolving business scenarios, enterprises also enjoy a competitive advantage when data is accessed quickly.
Based on the report by interviewquery.com, there is a 40% increase in data engineering interviews in the last year, whereas data scientists interviews have increased only 10% vis-à-vis an 80% increase in the past year.
What is Data Engineering?
While Data science requires Math and Statistical background, Data engineering requires technical knowledge as the role has the responsibility to build data pipelines. Data engineering has a broader aspect as it comes to various job titles. Different organizations offer different titles for data engineers. The main objective of a data engineer in an organization is to bring a consistent and organized flow of data to help in data-driven decisions such as:
- Performing exploratory data analysis
- Training of machine learning models
- Populating data into an application
Various Roles of a Data Engineer
The different job roles for a data engineer are Generalists, Database-centric Engineer, and Pipeline-centric Engineer.
Data Science Generalist has the expertise in data science life cycle. They have the capability of communicating the business insights to the leaders and have the expertise in business intelligence tools such as SQL, Tableau, and the design and optimization of machine learning algorithms.
Database Engineers need to have a combination of engineering and data science skills and have the skillsets to develop and maintain data architectures. They look after the collection of data and the conversion of raw data into usable data. The skillsets include SQL/NoSQL, Java, AWS, Scala and the ability to deal with Big Data.
ML Ops Engineer creates data pipelines to organize the validation, transformation, movement, and loading data from the data source to the final destination.
Responsibilities of a Data Engineer
A data engineer is mainly an IT personnel whose primary responsibility is to extract data for analytical and operational uses. Data engineers combine, integrate, and clean data so that it can be used for analytic applications.
The data handled by a data engineer varies according to the size of the organization. The larger the enterprise, the complexity of the analytics architecture also increases. Some of the industries such as financial, retail, and healthcare services are more data-intensive and dependent on data.
Usually, data engineers work closely with the data science team to improve the transparency of data that enables businesses to make reliable and right business decisions. More precisely, data engineers create data frameworks, data warehouses, and data pipelines for data scientists that help them to conduct complex data analysis.
The data engineers provide data to the data scientist in usable formats as they work on both unstructured and structured data. They should understand the various aspects of data architecture and its applications to handle both structured and unstructured data.
Data Engineering Skills
The skills expected from data engineers are more of software engineering skills. However, the focus should be on developing the skill sets such as general programming concepts, distributed systems and cloud engineering, and databases. Python is the topmost in the programming languages, which is a must for this profile. Other languages include R, SQL, Scala, C, etc.
They should have an understanding of data lakes and data warehouses including how these work. They have to be well-versed with business intelligence platforms through which the connections with data lakes, data warehouses, and other sources of data can be established. Other competencies include Apache Airflow, Hadoop, Cloud Infrastructure, etc.
Evolving Role of Data Engineers
The adoption of big data technologies across the industries leads to an increase in the growth of data engineering roles. The rapid evolution of data engineers happened in recent years and hugely because of the automation of business intelligence tools.
Business analytics platforms in modern times are equipped with completely automated or semi-automated tools. With automated tools, a data engineer helps his/her team member to work efficiently.
The fact is that the data science field is expanding into sub-disciplines such as machine learning, data storytelling, visualization, etc. AI and neural networks are also becoming popular in various industries like healthcare, the automobile industry, information security, and finance. All these changes in different fields will increase the demand for clean and transformed data provided by data engineers. Information security is a key element of data engineering, which necessitates the need for competent data engineers for keeping the enterprise data safe.
Building Transformational Skills
Based on the hiring parameters prevailing in the industry, the data engineers should have the educational qualification of M. Tech/ MCA/ M. Sc. Statistics or Economics/MBA/ B. Tech or equivalent qualification with a strong foundation in machine learning algorithms.
REVA Academy for Corporate Excellence-RACE offers MBA in Business Analytics (AICTE and UGC approved) that enables the data engineering aspirants to transform their careers. The program participants will get to work on real-time industry-grade projects combining Data Science, Data Engineering and ML-Ops and thus building full-stack skills to transform into an analytics professional who can build AI/ML products or projects.